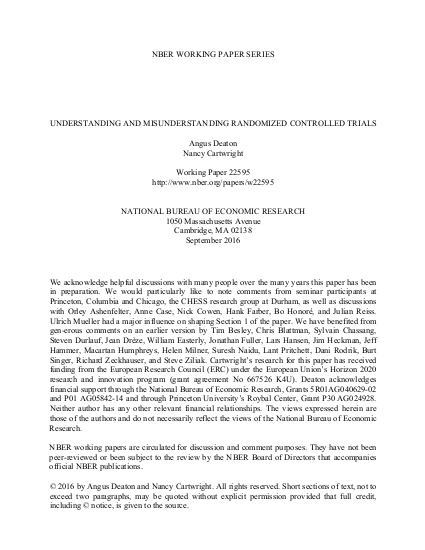
The use of RCTs is spreading in economics. They are seen as desirable for discovery and for generating evidence for policy. Yet some of the enthusiasm for RCTs comes from misunderstandings: that randomization provides a fair test by equalizing everything but the treatment and so allows a precise estimate of the treatment alone; that randomization is required to solve selection problems; that lack of blinding does little to compromise inference; and that statistical inference in RCTs is straightforward, because it requires only the comparison of two means. RCTs do indeed require minimal assumptions and prior knowledge, an advantage when persuading distrustful audiences, but a crucial disadvantage for cumulative scientific progress, where randomization undermines precision. It is hard to use them outside of their original context. Yet, once they are seen as part of a cumulative program, they can play a role in building general knowledge and useful predictions, provided they are combined with other methods, including conceptual and theoretical development, to discover not “what works,” but why things work. Unless we are prepared to make assumptions, and to stand on what we know, making statements that will be incredible to some, all the credibility of RCTs is for naught.